
Archives
Contribute
|
Technology - Quantifying Performance Using Human Function Signatures
|
|
Shawn McGuan 11/13/2006
Introduction
The more clearly you can see into the future, the better off you will be. As forward-thinking as modern design is, subjective measurement is still quite popular in classifying the performance of designs which will interface with people.
For "human engaged" products such as sport shoes, companies still rely on test subjects to provide performance measures on new designs, with the athlete running on the treadmill responding to queries about cushioning and stability by calling out subjective ratings. In the auto industry, human test subjects respond to the "feel" of the shift quality with descriptors like, "clunky", "mushy", etc. The golf industry relies on the feedback from highly skilled golfers which may or may not be appropriate for the average golfer who is the target customer. And the orthopedic industry still relies largely on clinical performance data or feedback from a small number of patients using the product for many years.
There are two inherent difficulties with these methods. The first is that they require a physical product prototype be manufactured. The second is that they are subjective and mutable. Now what if you were not required to manufacture the product in order to receive these measures? Better yet, what if the measures were from a test subject who would not fatigue, have a bad day or change in any way between design iterations? And finally, what if these measures were objective, even quantitative and could be compared against other evidence to create predictive, repeatable measures of product performance?
How are designs traditionally developed?
Virtual prototyping in the product development cycle has been utilized since the 1960's; starting with finite element analysis (1960's), multi-body simulation (1970's), computer-aided-design (1980's), and including variational analysis such as design of experiments and optimization (1990's). These methodologies allow the designer to examine the physical response of the component or system in its operating state before creating expensive physical prototypes. Until recently, these tools have been used largely on the product in isolation from the human component.
With the availability of modern, professional human simulation tools today, the question becomes how does one apply them in the product development process?
First, let's review the modern design process complete with virtual design/testing and physical design/testing components in Figure 1.
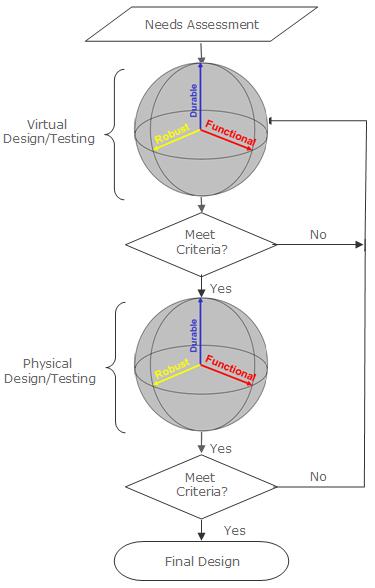
Figure 1. Modern product development process. The virtual design/testing component uses traditional CAD, FEA, multibody simulation to optimize functionality while maintaining good product robustness and durability. Once this is achieved a physical prototype is manufactured and physically to meet the same criteria.
In this process, a virtual product concept is developed using CAD and tested for durability with FEA and some functional performance using multibody simulation. Product sensitivity studies to assess robustness may or may not be performed at this stage. When the design is acceptable, a prototype is manufactured and physically tested on wear simulators, test rigs and with human subjects. If the design is deemed not acceptable, the process repeats itself starting from the virtual design/testing stage.
For human engaged products, the functional component in the virtual design/testing stage is very limited. In fact, in many cases, very little attention is paid to the functional component until the physical testing stage.
Wouldn't it be nice to consider the human?
With human engaged products, it might be a good idea to consider the human as early in the design process as possible. This would lead to deeper design insight, fewer prototype iterations and subsequently a better product delivered to the market faster. How could this be done?
The designer uses a four part process, first creating an appropriate human model, optimized to produce accurate consistent results without being overly complex. The model is validated for accuracy throughout the planned range of events. The third step is to develop a small set of key usage simulations that cover the range of product events. From these simulations, concise data measures are developed which characterize performance creating a "signature" which can be compared to new design iterations as well as to competitive products.
Let's consider a human simulation model which could "run" in a new sport shoe concept, or "swing" a new golf club concept, or even "climb stairs" with a new knee replacement system. Basically providing more information on the functional design aspect shown in Figure 1.
Take a golf club for example; in this case a certain shaft stiffness will only be optimal for a certain segment of the population. The signature of a specific club configuration could be the human power flow necessary to achieve a optimal swing. This power flow signature may then be used to indicate what type of golfer can use the product. Also, with the capability to produce this signature, golfers may be compared from an energetic level.
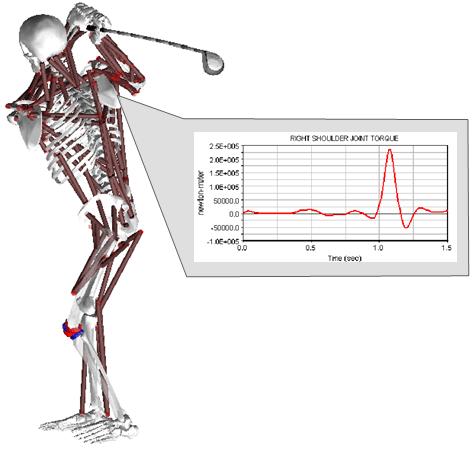
Figure 2. Musculoskeletal human model swings a golf club with a flexible shaft. Model also contains a total knee replacement in the left leg. Model reports data on comfort, energetics, loads on implant, stresses in shaft. These data comprise the signature of the golf club concept. The graph displays one aspect of the signature, the shoulder joint torque history for the swing.
In another example, instead of taking the time to build a new sports shoe prototype and then obtaining subjective ratings, sport shoe manufacturers have benefited from using human simulation to quantify performance while in the concept stage of development.
A human model, specifically a detailed foot and leg (see BRG Newsletter Volume 2) provides performance data on the engineering trade-off between shock attenuation and stability. In this case, the comfort signature could be the ground contact impulse felt through the body while the stability signature could be the loads on the lateral ligament complex in the ankle.
A baseline for the signatures could be derived from barefoot cases and simulations involving competitor shoes. This benchmark is then used to develop the optimal midsole for the appropriate sport and athlete size.
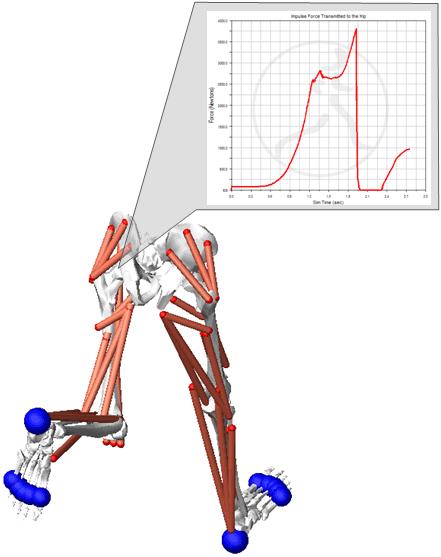
Figure 3. Musculoskeletal human model runs with ellipsoids (blue) representing paramaterized compliance elements. The mechanical properties of these elements are optimized for a variety of activities such as running, jumping, and lateral motion; providing data on an optimal configuration of a sports shoe. The signature for this event is the shock attenuation profile and the lateral ligament tension. The graph displays one aspect of the signature, the impulse curve of the ground reaction force as felt in the hip.
Other examples and the human centered design cycle will be discussed in the next issue.
(Shawn McGuan is a Member of the Biomechanics Research Group, Inc. based in San Clemente, CA. For further information, visit www.lifemodeler.com. )
|
You may also access this article through our web-site http://www.lokvani.com/
|
|